ㅣSymposium 1
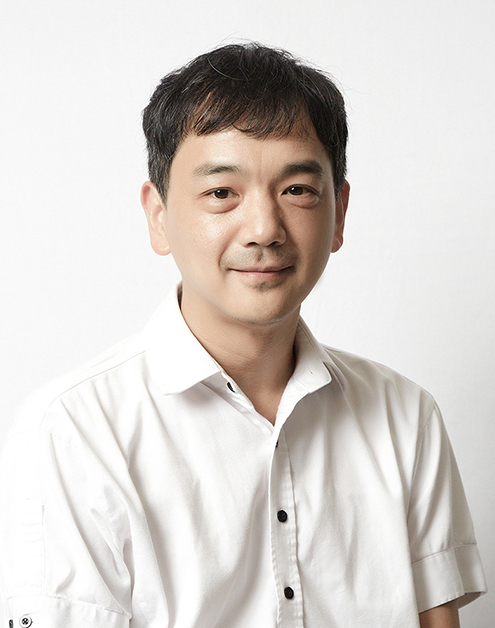
Prof. Sung-Phil Kim
Department of Biomedical Engineering, Ulsan National Institute of Science and Technology, Korea
Towards practical use of P300-based brain-computer interfaces
P300-based brain-computer interfaces (BCIs) read users’ intention primarily from the P300 component of event-related potentials (ERPs) by non-invasively measuring electroencephalography (EEG) and execute functions based on inferred intention. P300-based BCIs have advanced to support the activities of daily living of humans with paralysis. Recently, the applications of P300-based BCIs for daily activities in healthy people have been widely developed. Yet, there still remain many practical issues to be resolved to move P300-based BCIs in our real life. In this talk, I will present our recent work on potential solutions for some of those practical issues. Specifically, I will discuss how distractions in daily life can affect the BCI performance and potential solutions to deal with them. Also, I will talk about a feasibility to transfer a P300-based BCI built from pre-existing data to a new user, which may enable a “plug-and-play” BCI. I will discuss other issues too, including the necessity of daily calibration of BCIs and a solution to avoid it. I will wrap up the talk by discussing ways to facilitate practical applications of P300-based BCIs.
Biography
Sung-Phil Kim is professor of the Department of Biomedical Engineering at Ulsan National Institute of Science and Technology (UNIST). He is a director of the Brain-Computer Interface Lab at UNIST. Before coming to Korea, He was a post-doctoral researcher at Brown University until 2009. He received his PhD and M.S degrees in Electrical and Computer Engineering from University of Florida in 2005.
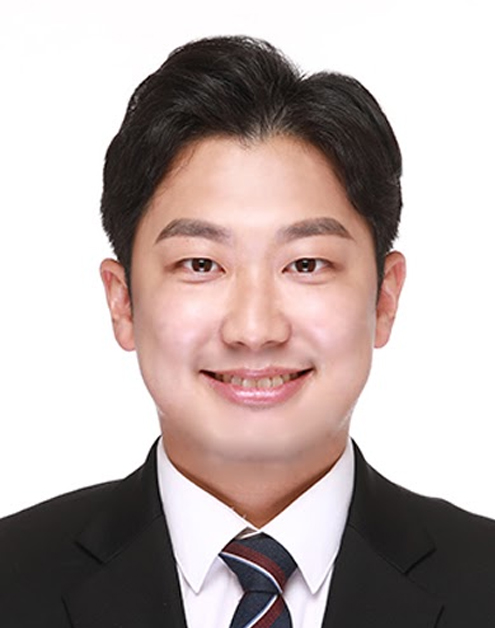
Prof. Ji-Hoon Jeong
Department of Computer Science, Chungbuk National University, Korea
Direct Brain Decoding Possible: Brain-Computer Interface and its Applications Using Intuitive Paradigms
Direct brain decoding represents one of the ways to establish a connection between the human brain and external machines. This concept facilitates two-way communication, utilizing the brain-machine interface (BMI). Specifically, EEG-based BMI has shown significant benefits in assisting patients with motor function recovery and has recently been validated for use in healthy individuals, owing to its ability to directly interpret human intentions. The exploration of neurolinguistic research using EEGs has provided a natural and intuitive mode of communication between humans and machines. In our study, we concentrated on EEG signals associated with speech imagery tasks, and our proposed deep neurolinguistic learning architecture successfully decoded neural languages. We evaluated the feasibility of accomplishing cooperative tasks based on BMI using various neural languages across eight subjects. We successfully demonstrated the intuitive BMI system in diverse scenarios, encompassing essential activities, collaborative play, and emotional interaction. These findings suggest the advancement in the BMI field, expanding the limits of intuitive, bidirectional brain-machine interaction.
Biography
Ji-Hoon Jeong received a Ph.D. degree in brain and cognitive engineering from Korea University, Seoul, Republic of Korea, in 2021. He is currently an Assistant Professor at the School of Computer Science, Chungbuk National University, Cheongju, Republic of Korea. His research interests include machine learning, brain-machine interface, and artificial intelligence.
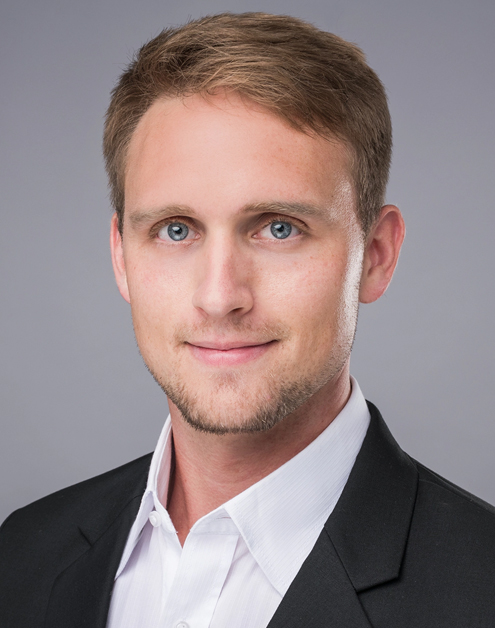
Dr. Alexander von Lühmann
Intelligent Biomedical Sensing (IBS) Lab, Technische Universität Berlin – BIFOLD, 10587 Berlin, Germany
Improving contrast for fNIRS Single Trial Analysis in the Everyday World: Progress and Challenges
Advancements in system design and signal processing have established functional Near Infrared Spectroscopy (fNIRS) as a cost-effective and practical modality for routine, increasingly unconstrained, and mobile brain imaging. The shift towards experimental studies and applications in dynamic, complex, and multisensory real-world environments offers numerous opportunities to progress research in physical and mental function and dysfunction. However, transitioning from well-controlled laboratory settings to the less predictable environment of the everyday world presents a range of challenges encompassing signal acquisition, processing, data fusion, and biomarker extraction. A key challenge in these contexts is extracting hemodynamics with sufficient contrast, such as distinguishing evoked from non-evoked activity and cerebral from non-cerebral activity. At the Intelligent Biomedical Sensing (IBS) Lab at Technische Universität Berlin, BIFOLD, we approach this issue by developing novel wearable neurotechnology and data-driven sensor-fusion methods that leverage multiple signal modalities. This presentation will examine recent advancements towards mobile brain-imaging using fNIRS. Subsequently, we will discuss the data science challenges that need to be addressed and demonstrate how we have begun to tackle them: Towards enabling the integration of these promising technologies into everyday environments.
Biography
Alexander von Lühmann is currently head of the “Intelligent Biomedical Sensing” research group at TU Berlin’s Machine Learning department and BIFOLD. He is also a visiting researcher at the Neurophotonics Center of Boston University (BU NPC) and the Lead Technology Advisor at NIRx Medical Technologies. Before this, he was the Chief Science Officer and R&D Director at NIRx for 2,5 years, a post-doc at Boston University, a visiting researcher at Harvard Medical School, and the Chief Technology Officer at Crely, a healthcare startup based in the US and Singapore. He received his PhD (Dr.-Ing.) with distinction in 2018 from TU Berlin, and his M.Sc. and B.Sc. degrees in Electrical Engineering from Karlsruhe Institute of Technology in 2014/11.